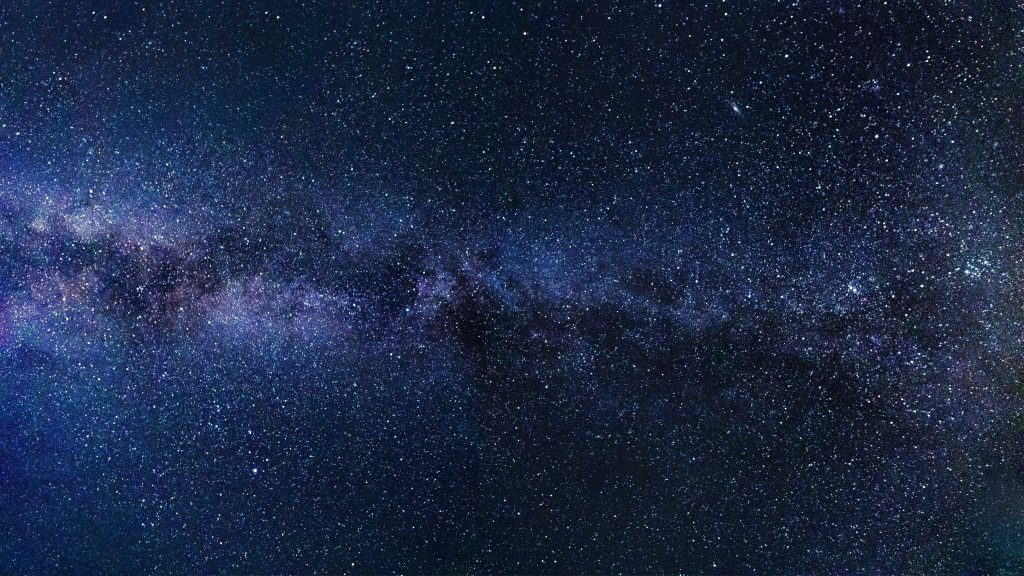
Procedural content generation (PCG) is a technique used in the gaming industry to create vast and diverse experiences for players. By employing algorithms and pre-defined rules, PCG can generate various elements of a game, such as terrains, levels, characters, and even narrative events. One of the most recent and exciting advancements in PCG is the incorporation of machine learning. This article explores the role of machine learning in procedural content generation and its implications for the future of the gaming industry.
Understanding Procedural Content Generation
Procedural content generation has been a powerful tool for game developers for several decades, allowing them to create expansive and dynamic virtual worlds. Traditionally, PCG relied on intricate algorithms, random seed values, and predefined rules to generate content. However, this approach often lacked the ability to create truly unique and refined experiences.
Enter machine learning, a subfield of artificial intelligence that aims to train algorithms to learn from data and make predictions or decisions without relying on explicit programming instructions. By leveraging machine learning techniques, PCG can now generate content that is not only procedurally generated but also tailored to individual player preferences.
The Blend of PCG and Machine Learning
The combination of PCG and machine learning introduces a new level of sophistication to game development. Instead of relying on fixed rules and algorithms, machine learning algorithms can analyze massive amounts of data, such as player behavior, preferences, and feedback, to generate content that is personalized and engaging.
For example, in a role-playing game, machine learning algorithms can learn from players’ past interactions and choices to create dynamic storylines that adapt to individual gameplay styles. As players progress through the game, the machine learning model continuously adjusts and improves its content generation process, resulting in a more immersive and tailored gaming experience.
Enhancing Content Diversity and Quality
Machine learning adds another layer of complexity to content generation by enabling the creation of diverse and high-quality game elements. By analyzing existing content and player feedback, machine learning models can learn the underlying patterns and structures that make certain gameplay elements popular or enjoyable.
For instance, a machine learning algorithm can examine thousands of game levels and identify patterns that evoke positive player responses. By understanding these patterns, the algorithm can generate new levels that embody the desired characteristics, resulting in more engaging and challenging gameplay experiences. This not only saves time for developers but also ensures a higher level of quality and diversity in generated content.
Fostering Game Design Innovation
The marriage of PCG and machine learning opens up new possibilities for game design innovation. With machine learning algorithms at the helm, game developers can experiment with unconventional and unique design ideas that would have been challenging or time-consuming to implement manually.
Furthermore, machine learning can assist developers in optimizing game balance and difficulty curves. By analyzing gameplay data, machine learning models can identify areas where players struggle or lose interest, allowing developers to make informed adjustments to enhance the overall gameplay experience.
Potential Challenges and Ethical Considerations
While the integration of machine learning in PCG offers immense potential, it also presents challenges and ethical considerations. One challenge is ensuring that the machine learning models do not inadvertently create biased or discriminatory content. Careful monitoring and manual intervention are necessary to prevent the appearance of inappropriate or unfair generated content.
Additionally, maintaining transparency and explainability of the content generation process is crucial. Players should understand that their experiences are shaped by machine learning algorithms and that their data is being used to train these models. Open communication about data collection and usage can help foster trust and mitigate potential privacy concerns.
The Future Landscape of PCG and Machine Learning
As the gaming industry continues to evolve, the integration of machine learning in PCG will likely become more prevalent. Advancements in machine learning techniques, coupled with the increasing availability of data, will allow for even more personalized and engaging gaming experiences.
Moreover, the combination of PCG and machine learning can extend beyond gaming. Industries such as architecture, virtual reality simulations, and even art can leverage these techniques to generate dynamic and immersive content tailored to individual users.
Conclusion
Machine learning has emerged as a game-changer in procedural content generation. By incorporating machine learning algorithms into PCG processes, game developers can create more diverse, personalized, and innovative experiences for players. However, it is crucial to tackle challenges related to bias, transparency, and privacy to ensure a responsible and ethical application of these techniques. With the continuous advancement of machine learning and the ongoing evolution of the gaming industry, the future of procedural content generation looks incredibly promising.